2019
2018
2017
2016
2011
2008
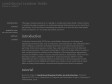
Conditional Random Fields

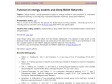
Dbn_Tutorial

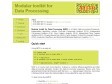
Modular toolkit for Data Processing (MDP)

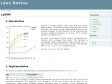
lasvm [Léon Bottou]

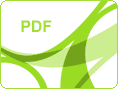
An Empirical Evaluation of Deep Architectures on Problems with Many Factors of Variation [PDF]

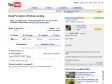
YouTube - Visual Perception with Deep Learning

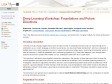
DeepLearningWorkshopNIPS2007 < Public < TWiki

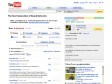
YouTube - The Next Generation of Neural Networks

2007
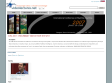
ICML 2007 - PRELIMINARY VIDEOS FROM THE SPOT

Elefant - What is Elefant

Amazon.com: "Machine Learning and Its Mathematics, Philosophy & Physics"

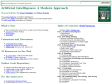
Artificial Intelligence: A Modern Approach

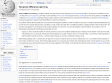
Temporal difference learning - Wikipedia, the free encyclopedia

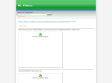
obousquet - ML Videos

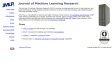
Journal of Machine Learning Research Homepage

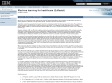
IBM Research | IBM Haifa Labs| Machine learning for healthcare (EuResist)

2006
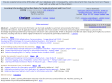
Learning Information Extraction Rules for Semi-structured and Free Text - Soderland (ResearchIndex)
